Data Science
Ph.D. Defense
Location
Integrated Science Center (ISC), Room 3291540 Landrum Dr
Williamsburg, VA 23185Map this location
Access & Features
- Open to the public
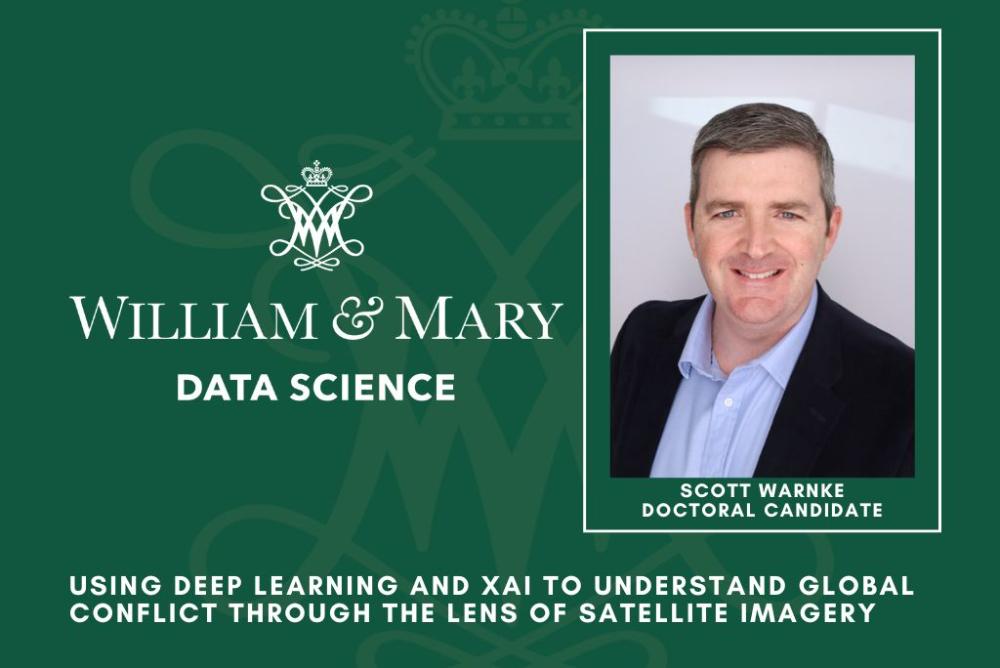
Social unrest, manifested through riots and protests, represents a persistent challenge for policymakers and urban planners worldwide. This dissertation demonstrates how high-resolution satellite imagery, combined with convolutional neural networks (CNNs) and explainable AI (XAI) methodologies, can uncover patterns associated with conflict occurrence in urban areas. First, we establish that a ResNet-based CNN can accurately predict the spatial distribution of riots and protests, achieving classification accuracies of up to 97% at a global scale by learning relationships between urban form and historic riot locations. Second, we explore the interpretability of these models using techniques such as Score-CAM, finding that deep learning often captures socio-spatial signals not readily explained by conventional GIS techniques. This observation underscores both the promise and limitations of existing XAI methods for satellite-based conflict analysis, where key features may not align neatly with standard land use or land cover categories. Finally, we address the spatial resolution requirements and semantic nature of these predictive features, revealing that critical cues typically appear at scales of 30–40 meters. By clustering and analyzing activation surfaces in the last convolutional layers, our framework identifies semantically meaningful feature classes, including open gathering spaces near prominent structures such as stadiums, markets, and religious sites. These human interpretable classes represent novel discoveries in the field of satellite imagery interpretation though deep learning. These insights not only demonstrate deep learning’s capacity to capture theoretically relevant indicators of unrest but also highlight the need for refined XAI approaches tailored to the unique challenges posed by satellite imagery. Collectively, this dissertation advances the fields of remote sensing, conflict prediction, and deep learning by establishing actionable pathways for leveraging CNNs and XAI to identify, interpret, and ultimately help mitigate the risks of urban unrest.
Sponsored by: Data Science