Arts & Sciences Events
[PAST EVENT] Jiangtao Kong, Computer Science - Defense
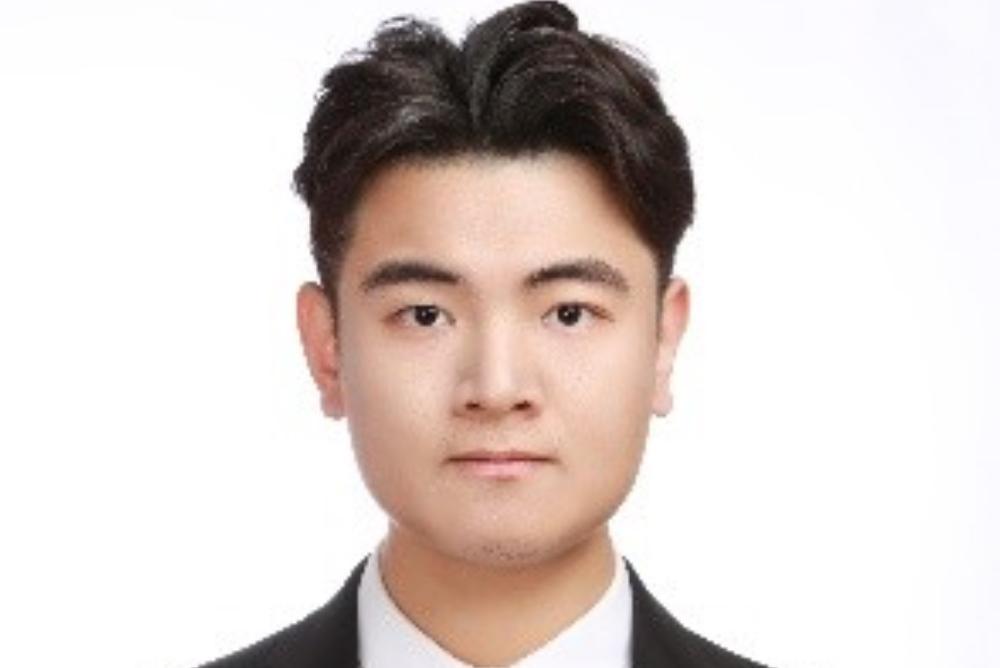
Abstract:
Incremental learning aims to enable machine learning systems to sequentially learn new tasks without forgetting the old ones. While some existing methods, such as data replay-based and parameter isolation-based approaches, achieve remarkable results in incremental learning, they often suffer from memory limits, privacy issues, or generation instability. To address these problems, we propose Recoverable Memory Bank (RMB), a novel non-sampler-based approach for class incremental learning (CIL). Specifically, we design a dynamic memory bank that stores only one aggregated memory representing each class of the old tasks. Next, we propose a novel method that combines a high dimensional space rotation matrix and Gaussian distribution to maximally recover the features of previous tasks from aggregated memories in the dynamic memory bank. After that, we use the recovered features together with the features in the current task to optimize the classifier. To balance the performance of our model between the current task and previous ones, we also adopt meta-learning to generalize and update the model, which promotes its capability of learning the current task effectively. Finally, the evaluation results on multiple benchmark datasets demonstrate that the proposed RMB significantly outperforms existing non exemplar-based methods in terms of accuracy. Importantly, it can achieve comparable performance to some exemplar-based methods.
Bio:
My name is Jiangtao Kong, a second-year cs master's student under the supervision of Dr. Huajie Shao at William & Mary. My research area is Deep Learning. Specifically, using the “memory mechanism” to make neural networks can learn new embedded information in the ever-changing open world.