A&S Graduate Studies
[PAST EVENT] Sunil Manandhar, Computer Science - Dissertation Proposal Announcement
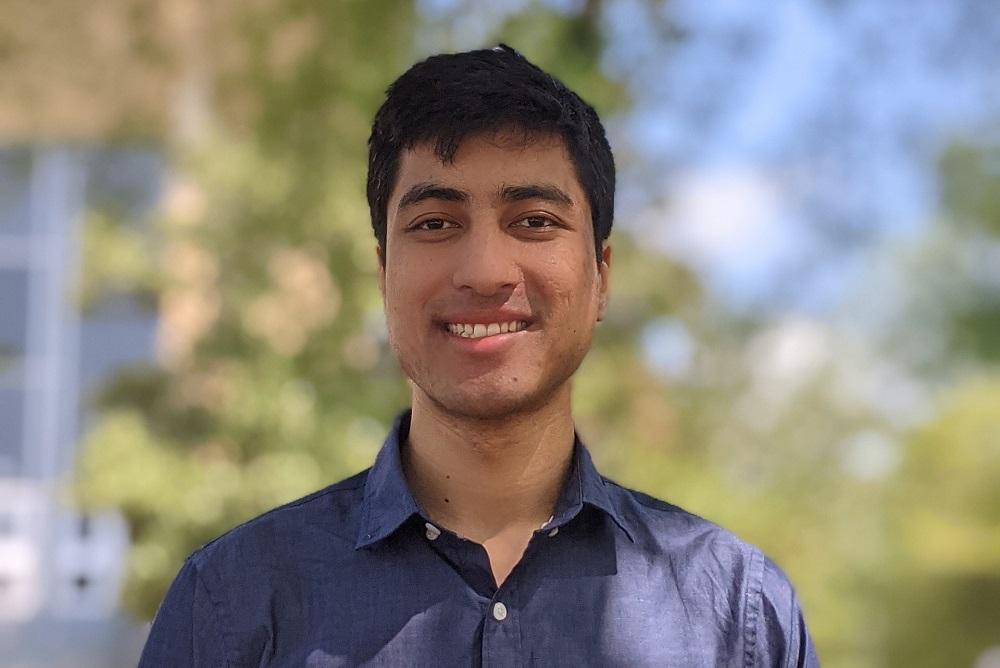
Abstract:
There has been a massive shift towards the use of IoT products in recent years. While companies have come a long way in making these devices and services easily accessible to the consumers, very little is known about the privacy issues pertaining to these devices. In this dissertation, we focus on evaluating privacy pertaining to commodity-IoT devices by studying device usage behavior of consumers and privacy disclosure practices of IoT vendors. Our analyses consider deep intricacies tied to commodity-IoT domain, revealing insightful findings that help with building automated tools for a large scale analysis.
We first present the design and implementation of Helion, a framework that generates natural home automation scenarios by identifying the regularities in user-driven home automation sequences, which are in turn generated from routines created by end-users. We hypothesize that smart home event sequences created by users exhibit inherent semantic patterns, or naturalness that can be modeled and used to generate valid and useful scenarios. To evaluate our approach, we first empirically demonstrate that this naturalness hypothesis holds, with a corpus of 30,518 home automation events, constructed from 273 routines collected from 40 users. We then demonstrate that the scenarios generated by H?lion seem valid to end-users, through two studies with 16 external evaluators. We further demonstrate the usefulness of H?lion’s scenarios by addressing the challenge of policy specification, and using H?lion to generate 17 policies that help to improve security/safety and privacy with minimal effort.
We then perform a systematic and data-driven analysis of the current state of smart home privacy policies, with a particular focus on three key questions: (1) how hard privacy policies are for consumers to obtain, (2) how existing policies describe the collection and sharing of device data, and (3) how accurate these descriptions are when compared to information derived from alternate sources. Our analysis of 596 smart home vendors, affecting 2, 442 smart home devices yields 17 findings that impact millions of users, demonstrate critical gaps in existing smart home privacy policies, as well as challenges and opportunities for their automated analysis.
Having laid out the groundwork to understand privacy challenges associated with commodity-IoT usage, we propose our research plan for automated compliance verification. We describe the importance of building tools that can automatically extract information and query complex documents (e.g., privacy regulations and privacy policies) to allow reasoning based on the regulatory requirements. We discuss possible ways in which the frameworks can be used to help reduce manual effort that goes into verifying compliance. Altogether, this dissertation provides insights related to privacy of commodity-IoT device usage that can be useful for all stakeholders i.e., vendors, researchers, and consumers. Furthermore, we develop several techniques that help with mitigating privacy and security risks.
Bio:
Sunil Manandhar is a PhD Candidate in the Department of Computer Science at William & Mary. His Ph.D. advisor is Prof. Adwait Nadkarni. His research focuses on understanding privacy and security issues in emerging platforms such as IoT. His Ph.D. research appeared in IEEE S&P ’20, CODASPY ’19, and ACM TCPS ’21. Previously, he received his Bachelor of Science in Computer Science and Information Technology from Tribhuwan University, Nepal in 2014. He worked as a research intern at IBM TJ Watson Research Center, NY in the fall of 2020, and worked as a COVES fellow with Center for Innovative Technology (CIT) in 2021.