W&M Featured Events
[PAST EVENT] Du Shen, Computer Science - Oral Exam proposal
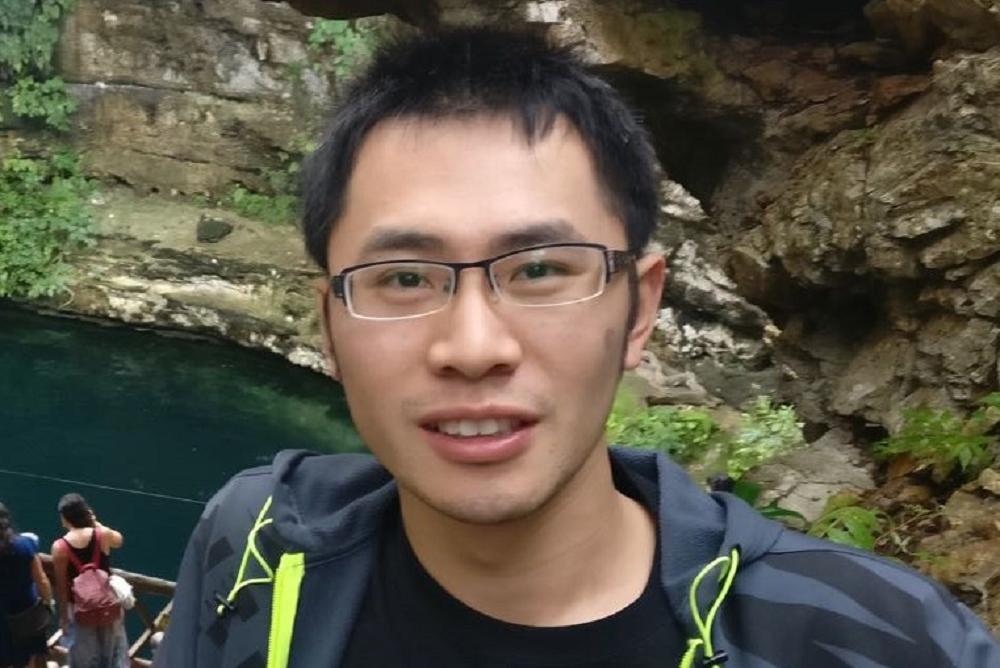
Abstract:
Heterogeneous architectures have become popular due to programming flexibility and energy efficiency. Heterogeneous architectures include GPU accelerators, and memory subsystems consisting fast and slow components. Achieving high performance for program on heterogeneous architectures requires sophisticated tools and applications. They either lack hardware support for fast memory component, or provide complex programming model, which puts extra burdens on compilers and programmers. However, existing tools either rely on simulators or lack support across different GPU architectures, runtime or driver version, thus providing insufficient insights.
In the first project, we develop DataPlacer, a profiling tool to provide guidance for data placement. We characterize a real heterogeneous system, the TI KeyStone II, whose memory system consists of fast and slow component, and the fast memory lacks hardware support. We develop a set of parallel benchmarks to characterize the performance and power efficiency of heterogeneous architectures. DataPlacer analyzes memory access patterns and provides high-level feedback at the source-code level for optimization. We apply the data placement optimization to our benchmarks and evaluate the effectiveness of HM in boosting performance and saving energy.
In the second project, we present CUDAAdvisor, a profiling framework to guide code optimization in modern NVIDIA GPUs. General-purpose GPUs have been widely utilized to accelerate parallel applications. Given a relatively complex programming model and fast architecture evolution, producing efficient GPU code is nontrivial. CUDAAdvisor performs various fine-grained analyses based on the profiling results from GPU kernels, such as memory-level analysis (e.g., reuse distance and memory divergence), control flow analysis (e.g., branch divergence) and code-/data-centric debugging. CUDAAdvisor supports GPU profiling across different CUDA versions and architectures. We demonstrate several case studies that derive significant insights to guide GPU code optimization for performance improvement.
Biography:
Du Shen has been working on his Ph.D. degree in the Department of Computer Science, William & Mary since Spring 2014. He is working with Dr. Xu Liu in the field of High Performance Computing. Before that, he obtained his M.S. in 2013 from William & Mary, and B.S. in 2011 from Nanjing University, China.