W&M Featured Events
[PAST EVENT] Daniel Highland, Computer Science - Thesis Defense
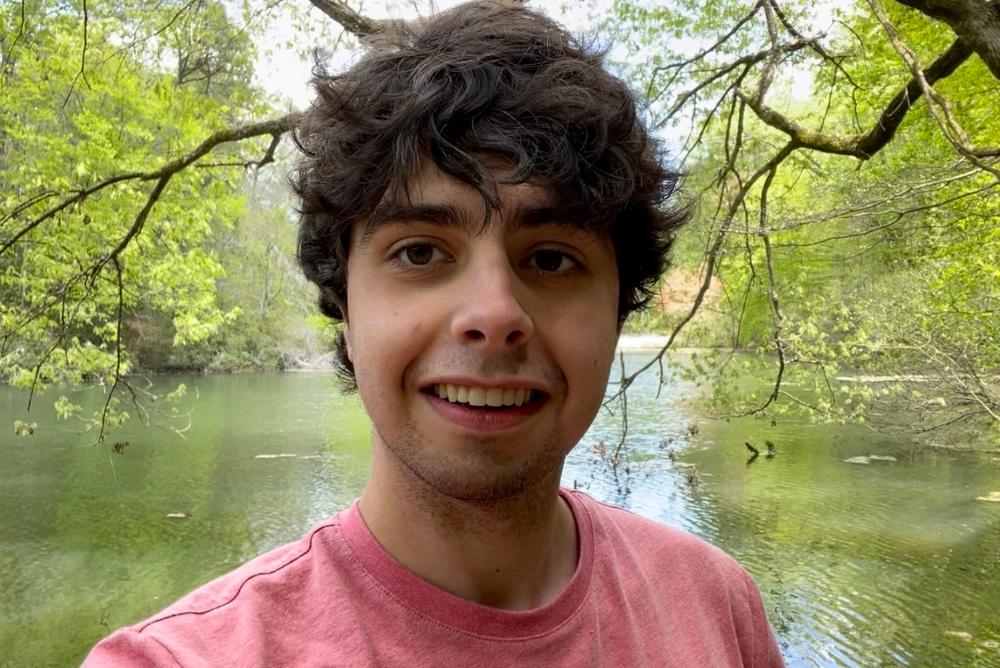
Abstract: Bacterial vaginosis (BV) is a common vaginal infection that can predispose patients to several complications, such as cervicitis. Like many illnesses, existing diagnostic methods face a trade-off between diagnostic confidence and lower costs. To help address this dilemma, we developed a number of deep learning models to evaluate the usefulness of different inexpensive diagnostic tests to better automate the diagnosis of BV. We first determined how best to utilize images of epithelial cells to diagnose BV according to the clue cell test of the Amsel criteria. We found that, in a single-image case, training a ResNet18 model on diagnostic results from molecular testing led to an 89% F1 score for binary diagnosis. We then tested if the addition of the other Amsel criteria by multi-layer perceptrons could improve these results, finding that adding only binary whiff test results led to a performance increase to an almost 91% F1. These results provide the first insight into how combinations of images and other test data under the umbrella of the Amsel criteria can best be used for reliable diagnoses. Future work should build on our methods and develop more comprehensive datasets for greater diagnostic confidence.
Bio: Daniel Highland is a 2nd year computational science MS student studying medical applications of machine learning under Dr Gang Zhou. Previously, he received a Bachelor of Science in chemistry at William & Mary, participating in the Wustholz spectroscopy lab and AidData's Eastern European Media Ownership project during his studies. Outside of university, he works to widen global access to finished pharmaceutical products as a spectroscopy intern for Family Health International 360.