W&M Featured Events
[PAST EVENT] Schmidt Family Distinguished Talk: Yunsi Fei
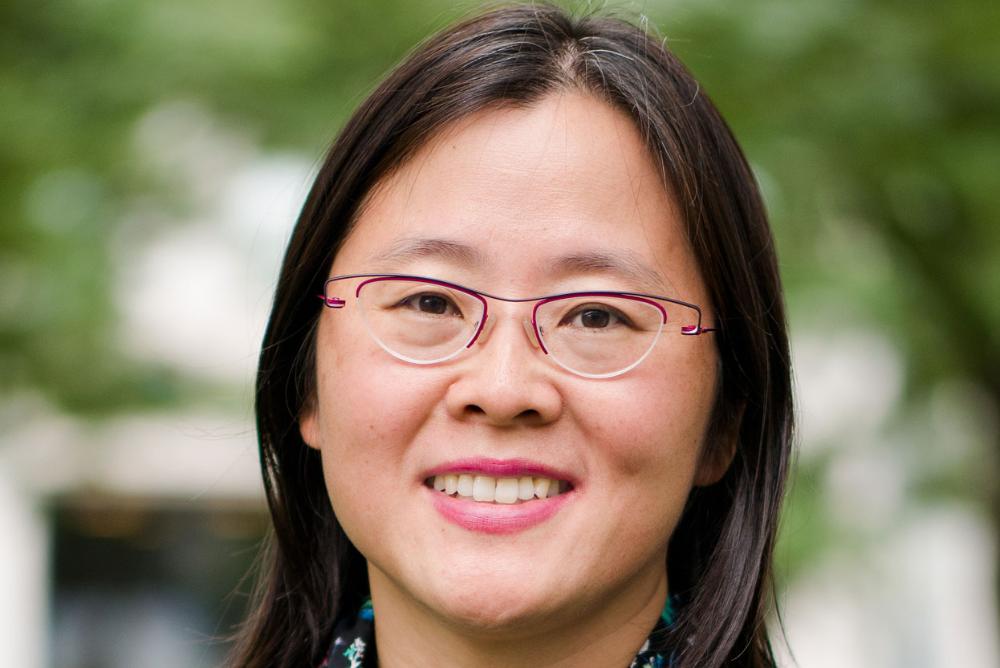
Side-channel for Security: Foe or Friend?
Abstract:
Side-channel leakage of a system execution contains rich inherent information about system operations and data. Over the past two decades, side-channel analysis has been conducted on various crypto systems for secret/key retrieval. In recent years, vulnerabilities of deep neural networks against side-channel attacks also become a serious security concern. In this talk, I will first introduce a comprehensive and effective reverse-engineering methodology for FPGA DNN accelerator soft Intellectual Property (IP) cores. Our method relies on schematic analysis and, innovatively, electromagnetic (EM) side-channel analysis to reveal the data flow and scheduling of the DNN accelerators. We demonstrate its application on the cutting-edge AMD-Xilinx Deep Learning Processing Unit (DPU). To the best of our knowledge, this research is the first successful endeavor to reverse-engineer a commercial encrypted DNN accelerator IP. Moreover, we recover DNN model architectures and extract model parameters via EM leakage. These outcomes pose a significant threat to real-world commercial FPGA-DNN acceleration systems.
On the other hand, based on the intuition that EM emanations of a DNN model inference may contain footprints of different input classes, we aim to leverage system side-channel signals for security protection. We propose a framework, EMShepherd, to capture EM traces of model execution, perform processing on traces and exploit them for adversarial example detection. Only benign samples and their EM traces are used to train the adversarial detector: a set of EM classifiers and class-specific unsupervised anomaly detectors. When the victim model system is under attack by an adversarial example, the model execution will be different from executions for the known classes, and the EM trace will be different. We demonstrate that our air-gapped EMShepherd can detect different adversarial attacks on a commonly used FPGA deep learning accelerator for both Fashion MNIST and CIFAR-10 datasets, effectively safeguarding the system.
Biography:
Yunsi Fei is currently a Professor of Electrical and Computer Engineering, and Associate Dean for Faculty Affairs of College of Engineering at Northeastern University. She directs the Northeastern University Energy-efficient and Secure System (NUEESS) laboratory. She received her BS and MS degrees in Electronic Engineering from Tsinghua University, China, in 1997 and 1999, respectively, and her PhD degree in Electrical Engineering from Princeton University in 2004. Her recent research focuses on hardware-oriented security and trust, side-channel attack analysis, protection, and evaluation, deep learning security, and secure computer architecture and heterogeneous systems. Currently she is the site director for an NSF Industry University Research Cooperation Center - Center for Hardware and Embedded System Security and Trust (CHEST), and actively engaging with industry partners to address security needs arising in their applications and products. Her research group has received several best paper awards, including AsiaCCS2023 and ICCD2017, with extensive support from both government agencies and industry.
This talk is open to the public and a part of the distinguished speaker series sponsored by the Schmidt Family.