A&S Graduate Studies
[PAST EVENT] Atiqur Rahman, Computer Science - Ph.D. Dissertation Defense
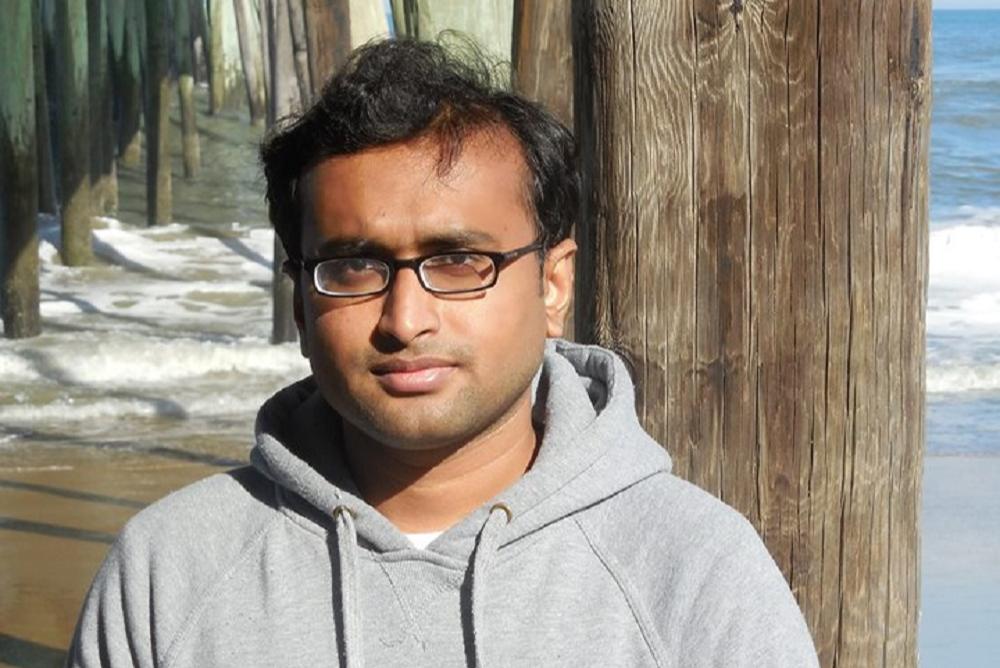
Abstract:
Spatiotemporal and image data is growing rapidly in diverse domains with the advancement of technology. Advancements of machine learning and computing resources allow us to analyze the data for various purposes such as classification, prediction, clustering, and anomaly detection. While machine learning often delivers impressively accurate results, its methods do not necessarily help humans gain a deeper understanding and real insights into a domain. The latter is, in particular, a concern in scientific research in natural sciences. Identifying characteristic features of phenomena of interest and patterns in measurement data is better aligned with the derivation of knowledge in the sciences. This makes combining and complementing feature and pattern mining with machine learning a very promising research direction. In this thesis, we explore four different problems in different domains where feature and pattern mining is essential for a better understanding of the analyzed data. The dataset analyzed contains spatiotemporal data where spatial information represented as an image.
First, we study time series data in cellular biology to identify features that help distinguish different developmental stages of Xenopus laevis. While the actual data lead us to consider entropy of a discrete time Markov chain that is derived from a discretized time series of measurement data, we also conducted an in-depth simulation study to understand that entropy and related properties such as trace, and 2nd largest eigenvalue of a DTMC respond to periodicity in the time series. The latter is even influenced by autocorrelation.
Second, we analyze the spatial aspects of cellular biological data in the feature and pattern mining process. To capture the spatial aspect, we register calcium images (imaged calcium activity of neural plates of Xenopus embryos at the neural plate stage) with a cellular resolution to a global coordinate system, the so-called template neural plate. In the template neural plate, the cell is represented by an aggregate feature extracted from the calcium activity time series of the cell. Our analysis suggests that calcium activity is spatially stochastic and does not correlate with embryonic gene expression.
Third, we study feature mining in image data, particularly to measure the degree of corrosion from images captured from a piece of aging infrastructure. We first apply deep learning-based image segmentation technique to identify and segment corrosion from the captured image to quantify the amount of corrosion. However, this is only a first step to evaluate the health of the infrastructure. Additional information such as the degree of corrosion is needed, which we propose to measure in this study with a particular feature.
Last, we analyze image data that is obtained from camera traps obtained in a forested area to recognize deer activity. The goal is to estimate the number of deer visiting the location over time. In addition to that, we compute features such as the relative height of the deer to analyze deer growth over time. To estimate the deer visit rate, we apply deep-learning based object detection and classification techniques to detect deer in image data and classify deer into three categories: male, female, and baby deer. To measure the relative height, we segment the detected deer images and then compute the height from the segmentation. This study contributes to an automated data analysis pipeline for ecologists to further explore deer behavior and environmental factors that affect deer activities and the growth of deer.
Bio:
Atiqur Rahman is a Ph.D. candidate at William & Mary, advised by Dr. Peter Kemper. He received a Bachelor degree in Computer Science and Engineering from Bangladesh University of Engineering and Technology in 2009. His research focuses on feature mining on spatiotemporal data.