Biology Events
[PAST EVENT] Atiqur Rahman, Computer Science - Ph.D. Dissertation Proposal
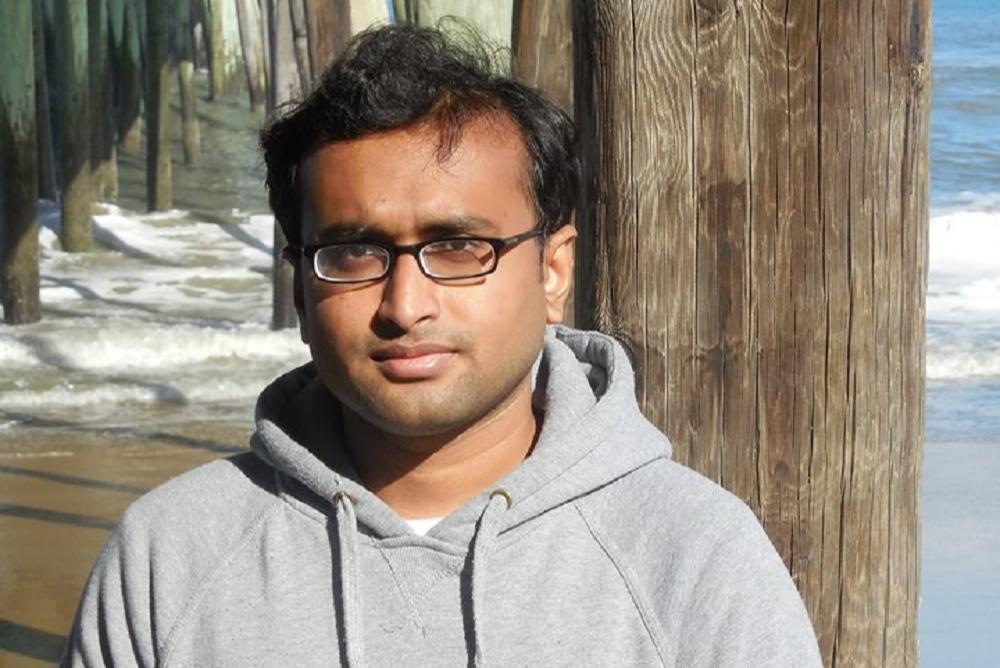
Abstract:
Spatiotemporal and image data is growing rapidly in diverse domains with the advancement of technology. Advancements of machine learning and computing resources allow us to study these data for various purposes such as classification, clustering, prediction, and anomaly detection. But it is not always intuitive to get the insight of the data from the machine learning algorithm applied, thus not always meeting the requirement of various domains where insight of the data is more important than the classification and prediction task. Meaningful feature and pattern mining can achieve that. In this research, we explore four different problems in different domains to get the insight of the data using feature and pattern mining. The problems are chosen from three different domains which include cellular biology, ecology, and infrastructure health monitoring. The dataset contains spatiotemporal data where spatial information is represented as image. In the first problem, we explore temporal feature mining in cellular biology and analyze what aspects of the data those features represent. Study suggests that entropy of discrete time Markov chain (DTMC) of discretized time series can distinguish different development stages of Xenopus laevis. In our analysis, we find that entropy and related properties such as trace and 2nd largest eigenvalue of DTMC can recognize the extent of periodicity in a time series. We also observe that autocorrelation in the time series is recognized by 2nd largest eigenvalue. In the next problem, we include the spatial aspects of the cellular biological data in the feature and pattern mining process. In order to capture the spatial aspects, we register calcium images (imaged calcium activity of neural plates of Xenopus embryos at neural plate stage) with cellular resolution to the template neural plate. Our analysis suggests that calcium activity is spatially stochastic in nature and also do not correlate with embryonic gene expression. In the next problem, with the collaboration of Bentley Systems, we propose a methodology to measure the degree of corrosion from the captured image of aging infrastructure. We first apply deep learning-based image segmentation technique to identify and segment corrosion form steel bridges and tunnels. Then from the segmented area with corrosion, we measure the degree of corrosion with a proposed feature based on the image color channels. In the last problem related to image data analysis, we aim to classify male, female and baby deer from the camera trapped images and to estimate population in the area image taken with the end goal to identify deer activities.
Bio:
Atiqur Rahman is a Ph.D. candidate at William & Mary, advised by Dr. Peter Kemper. He received a Bachelor degree in Computer Science and Engineering from Bangladesh University of Engineering and Technology in 2009. His research focuses on feature mining on spatiotemporal data.