Data Science
[PAST EVENT] CS Colloquium Talk: Daniel Dunlavy
Access & Features
- Open to the public
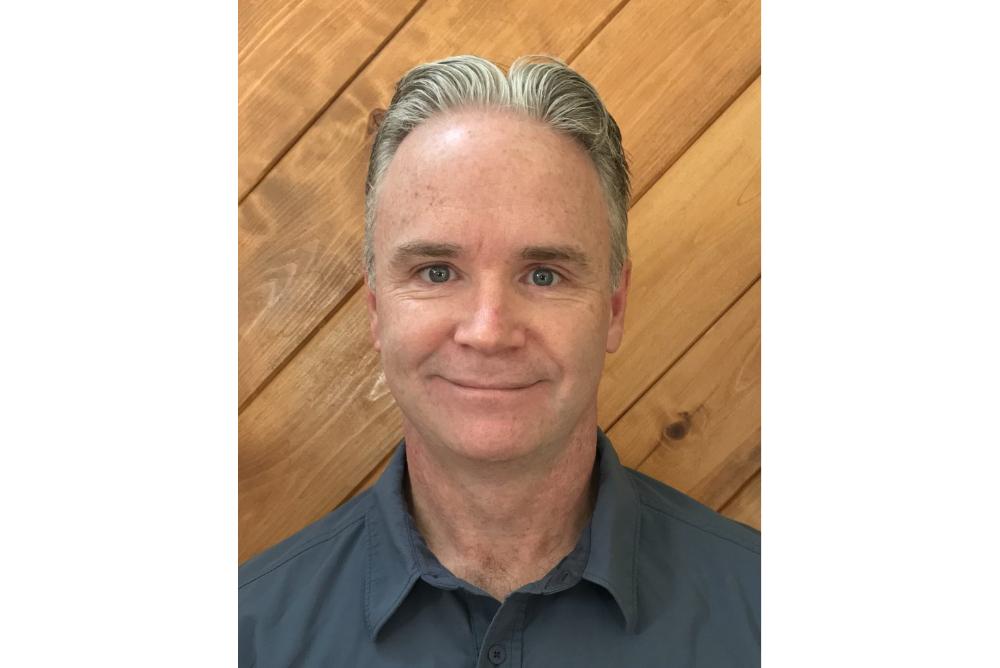
Title: Low-rank Tensor Decompositions for Count Data
Abstract: Low-rank tensor decompositions are useful in many data science tasks, such as data compression, noise reduction, pattern extraction, and data imputation. In many applications, the data consists of integers representing count values. In this talk, I will present an introduction to low-rank tensor decomposition, some of the challenges of working with tensor count data when computing decompositions, and how we can address these challenges in practice. Furthermore, I will describe several recent advances associated with low-rank decompositions of tensor count data, including topics on data modeling, computation, error analysis, and regression modeling.
Bio: Danny Dunlavy is a Distinguished Member of the Technical Staff in the Machine Intelligence and Vis Department of the Center for Computing Research at Sandia National Laboratories in Albuquerque, NM. His research interests include numerical optimization, numerical linear algebra, machine learning, data mining, tensor decompositions, graph algorithms, text analysis, parallel computing, and cyber security.
Sponsored by: Computer Science Department
Contact
[[oscarch]]