W&M Featured Events
[PAST EVENT] Thesis Defense for Aaron Harris, Computer Science
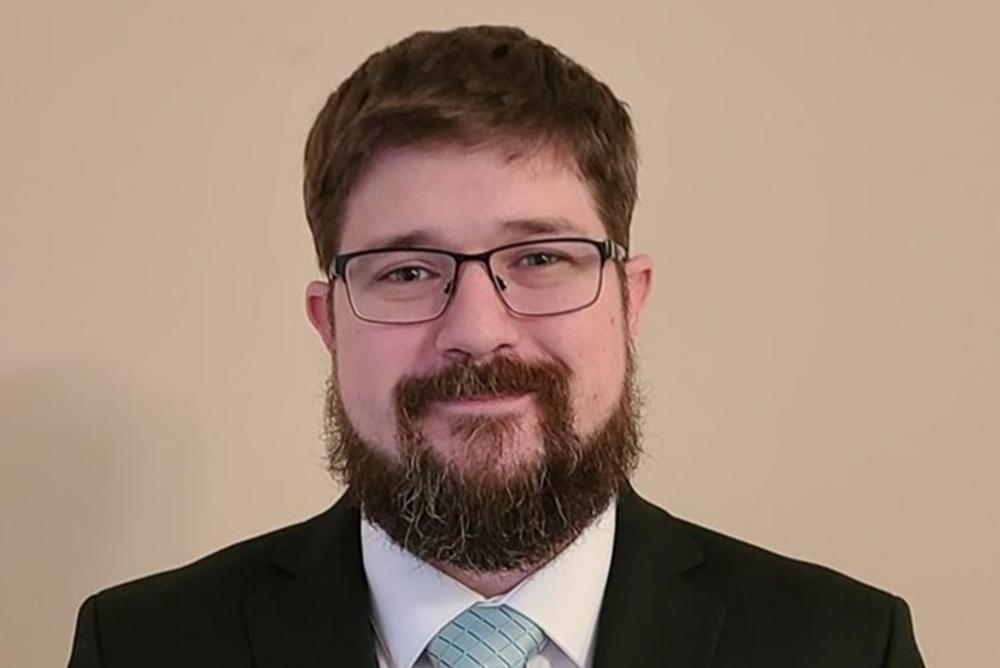
Abstract:
Considerable research has been performed with regard to using text-to-text machine learning methods to perform various software engineering tasks. At the same time, contrastive learning has shown promise in other modalities, such as computer vision-related problems, and has been explored to some extent in terms of limited software engineering tasks. We demonstrate that contrastive loss, on its own, is insufficient to surpass current baselines for these tasks; however, we note that there is a high degree of orthogonality in the results from existing and contrastive models.
We show that when our contrastive method is used as an additional transfer learning step in the training process, the results contain a large portion of the overlap between the distinct models, as well as producing new positive results, effectively capturing the majority of the results from the distinct models and increasing overall model accuracy. By employing this method, we are able to exceed the baseline accuracy of four software engineering tasks by varying margins, ranging from 5% to 262% during single beam tests, with minor improvements at selected other beam sizes, in both single- and multi-task training strategies.
Bio:
Aaron Harris is an MS/PhD student in Computer Science at William & Mary advised by Dr. Denys Poshyvanyk. His research interests include software engineering and neural networks.
Sponsored by: Computer Science